The Merits of Quantitative Research
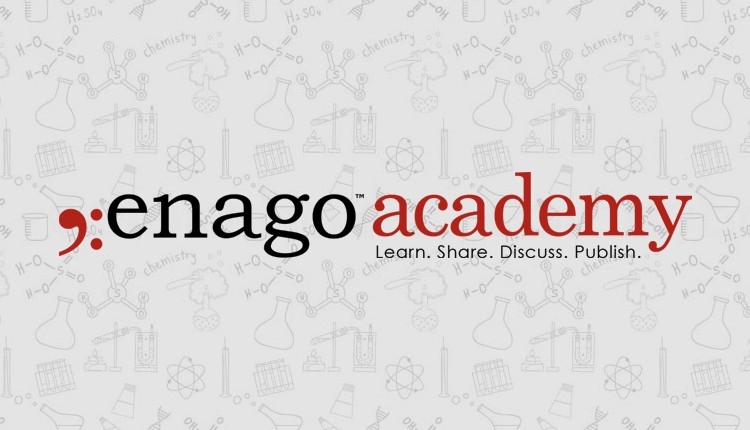
A Scientific Approach
Quantitative research methods are considered to be the preferred approach to academic research, with infrequent allowances for qualitative methodologies to be utilized when the research subjects (more often than not, human subjects) refuse to be codified into a robust analytical construct. As the name implies, quantitative research produces numbers that facilitate measurement, assessment and inference.
Hypothetico-Deductive
Advocates argue that the greatest strength of quantitative research is the clear grounding in theory: hypotheses are developed to test variants on established theories in order to refine them based on those identified variables. This doesn’t prove the established theories but rather improves them with additional datasets that support or align with the original results.
Using a sample population that may be much larger than for a qualitative study, data can be collected fairly quickly using survey tools via email or telephone, and the availability of statistical software allows for precise and accurate data analysis. The potential for research bias is limited to design of the study, design of the survey tool, and interpretation of data, as opposed to the broader risk of observer bias in a qualitative study.
The Predictive Power of Statistics
Since quantitative research data is delivered in numerical form, we can apply statistical tests to that data to produce descriptive measurements that can then be used to make predictions within the confines of the degree to which the sample population can be considered to be representative of a larger population. Starting with basic descriptive measures such as mean, mode, median, and standard deviation, we can establish a basic data framework before moving on to more inferential tests such as t-tests, ANOVAs, and multivariate measures such as multiple regression calculations (MRC).
The degree to which the results can then be generalized into a broader inference will depend on the almighty p-test that is used to determine the statistical validity of the data. Provided that the p-value is less than 0.05, the chance that the data was the result of chance is less than 5 percent.
Irreproducibility And False-Positives
The ability to delineate specific variables in the development of a hypothesis provides quantitative research with an inherent reproducibility. Since you’re testing variants of an established theory rather than proposing a new theory, researchers that attempt to validate your study with all the same protocols in place, should be able to replicate your results. When that doesn’t happen, the protocol is examined to verify that it matches with the original, and if that checks out, the original dataset should be next on the list, assuming, of course, that the authors of the study you are attempting to validate are willing to share that data.
If the original study were based on a false positive, where data analysis generated statistical significance for something that wasn’t really there, the likelihood of being able to replicate those results would be slim to none, unless you were able to map out the statistical analysis step-for-step.