How Significant is Statistical Significance?
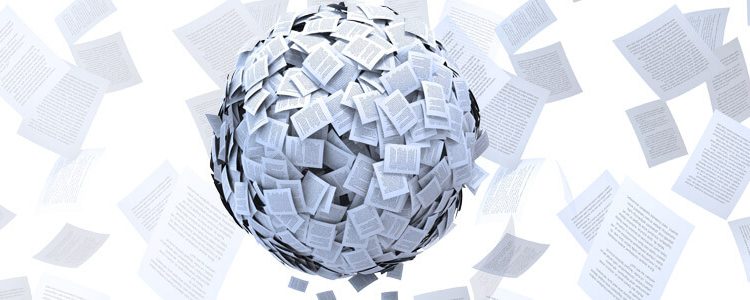
A problem of reproducibility has begun to emerge among researchers around the world. As scientists study increasingly complex systems with multiple variables, there will certainly be variation in observed effects. But more importantly, when seeking to understand scientific research produced by a peer, it is important to understand how statistics were used in their hypothesis testing. Commonly, scientists use p-value in statistical hypothesis testing to indicate statistical significance. But what happens when people stretch their data to observe a significant result? Such p-hacking, or cheating on p-value calculations, surely has contributed to the emerging problem of scientific reproducibility. There is a growing gap between the true meaning of p-value and how it is interpreted by non-scientists and scientists alike. Among under-funded and overworked researchers, the quest for statistically significant findings has resulted in poorly conducted data analyses that misrepresent the value of research outcomes.
P-value As a Measure of Significance
As a statistical tool, the p-value was originally designed as a way to measure the strength of evidence in support or against a hypothesis. Indeed, the p-value approach to analyzing data was developed to determine the probability that some intervention or treatment had no effect or makes no difference. This question of no effect is termed the null hypothesis. Indeed, scientists are often taught during their training the hypothesis is the foundation of all research – and at its most basic level, the lack of any difference between groups represents the null hypothesis.
When it was first developed, the p-value was used to identify statistical significance. When considering the probability of outcomes ranges from 0 to 1, a value of 0.05 seemed strenuous enough. If something happened by chance only in 1 out of 20 times (or at a probability of 0.05), then that something must be significant, right? But alas, a p-value <0.05 was only arbitrarily established as a general rule to guide statistical analyses.
It is true that a lower p-value indicates a reducing likelihood that chance accounted for an observed effect, but it is also important to remember that p-value does not indicate if something is true, but rather that there is a certain level of evidence against the null hypothesis. That is to say, a smaller p-value indicates a higher likelihood of rejecting the null hypothesis of no effect. When designing an experiment, it is important to remember that p-values are a reflection of the size of the sample population and how widely or normally such data are distributed. Therefore, in research hypothesis testing, to truly improve the confidence in the relevance of an observed effect, a young researcher would be wise to have a large sample size. Similarly, in understanding scientific research, it is important to be critical of the statistical methods used in studies.
Troubles in P-value Paradise
The aforementioned “reproducibility problem” has caught the attention of both funding agencies and journals. While many factors account for such errors in reproducibility, the most under-reported cause is simply flawed analyses. While most scientists make genuine efforts to faithfully design experiments and record data, some simply misunderstand the meaning of p-value. Rather, in the quest to ensure that their data are significant (and thus, “meaningful” in the minds of improperly trained biomedical researchers), P-hacking has increased in prevalence. At its most basic level, p-hacking reflects the practice of arbitrarily omitting only some data or running all types of statistical tests until a significant p-value is obtained. Such practices, in which scientists cheat on p-value, can result in substantial bias in the literature. Nevertheless, meta-analyses can be used to detect p-hacking and how the practice by certain authors contributes to data skewing.
Statistical and Meaningful Significance
Ultimately, why does statistical significance matter? When some scientists stretch their data while p-hacking, the reliability of p-value can be undermined from a purely social perspective. However, the biological meaning of statistical significance is not the same as clinical importance.
Indeed, p-value only indicates the likelihood that an event is due to chance rather than due to the presence of an actual event. One way to circumvent the difficulties in presenting data is to simply present data and leave the interpretation of its significance up to the reader. Regardless, it is important to be transparent and thorough in reporting how statistical methods were used. In doing so, reviewers can catch errors or suggest an improved method. Additionally, researchers can consider working with biostatisticians, who are often made available through research universities to aid scientists in their work
Ultimately, in the quest to uncover the nature of the world around us, scientists use hypothesis testing and p-values to assess the importance of their observations. In understanding scientific research, scientists must be both aware of the p-value of a finding, but also of potential cheating in calculating the p-value. While p-hacking exists, it may simply be the result of poor statistical methods rather than dubious efforts to present worthwhile data. As always, researchers benefit from the care and transparency in all areas of their work, including statistical analysis.