Responsible AI: Ensuring fairness and transparency in data science reporting
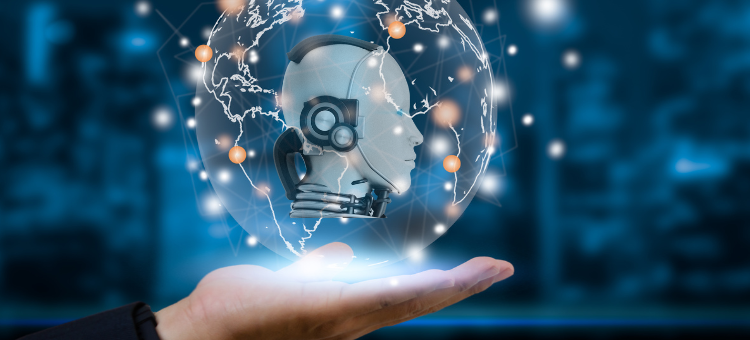
Overview
In today’s world, Artificial Intelligence (AI) and data science have integrated into numerous aspects driving decision-making processes in different industries like healthcare or finance while also impacting market trends or transportation systems worldwide. To those ends relying on AI, algorithms have become mandatory to tap into meaningful insights from a massive amount of data; however regulation may lead technological innovation down to problematic paths. The rise of responsible AI implies ensuring equity within all cadres and enforcing clearness regarding scientific knowledge transmission. In this article, we will explore the importance of responsible AI, the potential risks of biased algorithms, and strategies for promoting fairness and transparency in data science reporting.
Understanding Responsible AI
The development and implementation of AI systems that prioritize ethical issues, fairness, and transparency is referred to as “responsible AI.” It involves designing algorithms that do not perpetuate biases, treating all individuals fairly, and making the decision-making process understandable and explainable. Responsible AI is crucial to prevent the reinforcement of existing societal biases and discrimination and to ensure that AI algorithms are accountable and trustworthy.
The Risks of Biased Algorithms
Large datasets play a critical role in training AI algorithms. Yet these very same datasets may harbor inherent biases that go unchecked leading to amplified outcomes that further perpetuate existing societal inequalities. Take for example the case of recruitment where an AI algorithm trained on biased historical hiring data inadvertently favors certain demographics over others leading to discriminatory outcomes. Unaddressed biases in software applications have far-reaching negative effects from reinforcing stereotyping cultures to unjust implications in areas such as credit approvals, resource allocation, or criminal justice systems.
Promoting Fairness in Data Science Reporting
Keeping data science reporting impartial requires the implementation of various tactics. The objective is to combat prejudices, promote diversity and reduce the potential harm brought by AI algorithms. In this write-up, we shall examine each strategy in detail.
Diverse and Representative Data:
Achieving fairness mandates the incorporation of diverse and representative datasets in AI model training. Enabling different demographics, perspectives, and backgrounds contributes significantly to generating fairer insights. By incorporating varied information sources, it’s less likely that biases may emerge over time inadvertently fostering skewed views on issues affecting certain groups. Inclusivity remains key when collecting relevant data; hence equal representation matters.
Bias Detection and Mitigation:
In order to ensure equality is upheld throughout AI development, it is crucial for data scientists to implement stringent measures that can detect and mitigate harmful biases within algorithms. Conducting regular assessments of models will enable them to identify potential sources where prejudices might arise; thus allowing them to implement effective countermeasures involving pre-, in-, and post- processing techniques. For instance, pre-processing involves modifying data before training the model by employing tactics such as reweighting or oversampling underrepresented groups while in-process aims at curbing prejudices during the learning phase by adopting a fair-awareness loss function; On the other hand, “postprocessing” methods work towards eliminating any skewed results generated by recalibrating or setting thresholds.
Interpretable and Explainable Models:
A fundamental component of diversity is incorporating transparency and explainability within artificial intelligence (AI) models. This development is paramount for responsible implementation since it permits users to comprehend how an algorithm yields its verdicts. A few methods to achieve this goal are through Explainable AI techniques. Rule-based systems or model-agnostic approaches provide valuable insights into deciphering how AI arrives at decisions while eliminating biases along the way.
Regular Auditing and Monitoring:
The ethical usage of AI systems requires that organizations take proactive measures which promote fairness and transparency for all users involved. Consequently, it is pertinent for regular checks on how algorithms perform since discrepancies may evolve due to changes in the data landscape or other variables. Furthermore, stakeholders from different backgrounds should participate in the evaluation process so their perspectives can be duly considered where necessary.
Ethical Considerations:
Ensuring equitable deployment of artificial intelligence (AI) systems hinges on incorporating ethics during their creation process i.e., the establishment of transparent ethical protocols centered around equitable distribution rights protection privacy concerns.
By implementing these strategies, organizations can work towards creating fairer data science reporting practices. However, it is important to acknowledge that achieving perfect fairness is challenging due to the complexity of societal biases and the potential limitations of AI algorithms. It requires ongoing efforts, collaborations, and continuous improvement to address biases and ensure fairness in data science reporting.
Transparency in Data Science Reporting
Here are some recommended approaches one might take in order to promote transparent practices throughout various forms of communication when examining data science:
- Open Data Sharing: The sharing of datasets and methodologies in an open fashion should be a top priority for researchers and organizations alike. Collaboration thrives when there is open data accessibility while also empowering algorithms to undergo independent scrutiny. Making AI model data available along with associated documentation and code ensures that verifications are accurate while identifying potential biases; it encourages constructive suggestions for improvements made transparently by the entire community-wise resulting in responsible practices being embraced within the field of data science.
- Algorithmic Documentation: Without comprehensive documentation of AI algorithms, transparency remains an elusive goal. That’s why it’s important to include critical details such as feature engineering techniques, training data, and model architecture in the provided documents. Such detailed analysis opens up the workings of the algorithm to external validation and review while affording better comprehension for stakeholders.
- Ethical Guidelines and Standards: Guidelines dictating the proper use of AI algorithms must be followed to uphold ethics during their creation and deployment. Principles including fairness, privacy protection, explainability along with avoiding causing any harm all must be present within those regulations. It becomes pivotal for regulatory agencies along with research organizations in addition to industry associations to establish said guidelines responsibly while enforcing them as well.
- Model Explanations and Interpretability: To promote transparency in AI models, it’s essential to offer straightforward explanations and interpretations. End-users must comprehend how a model arrives at its decisions, along with the factors contributing to those judgments. Local explanation techniques-identifying essential features for individual predictions-and global explanations-understanding overall model behavior-can help provide insights into the decision-making process. Making the model interpretable allows stakeholders to assess potential biases or unfairness present within AI algorithms, ensuring there’s built trust in this technology.
- External Auditing and Validation: To achieve maximum transparency in AI systems implementation, it’s advisable to carry out independent external auditing and validation procedures. Organizations and experts who are not directly involved with development should be engaged for this purpose so that they can scrutinize the algorithms, datasets as well as methodologies that inform data science reporting. External auditing provides a vital mechanism for detecting any inclinations towards bias while helping assess fair practices that meet ethical guidelines set out within their organization or industry sector. The verification process provided by external validation enhances transparency considerably because findings reveal the reliability and fairness of AI systems.
- Public Engagement and Education: It is of great importance to implement public engagement and education programs as integral components of transparency. These initiatives can encompass a few undertakings such as workshops, conferences, and campaigns with the objective to sensitize the public and encourage discussions between stakeholders inclusive of researchers, policymakers, and the general public. Public views should also be integrated in AI development processes.
Promoting transparency in data science reporting requires a collective effort from researchers, organizations, policymakers, and the public. By implementing these strategies, stakeholders can foster transparency, accountability, and responsible practices in the use of AI technologies. Transparency not only helps address potential biases and discriminatory outcomes but also enables continuous improvement and the development of AI systems that better serve society.
Challenges and Future Directions
While tremendous strides have been made towards ensuring transparency and fairness in data science reporting efforts are still underway with significant challenges persisting. A major hurdle encountered is the absence of standardized methodologies or metrics to gauge fairness which makes measuring biases an arduous task. Robust evaluation frameworks need building so biases are precisely identified as well as quantified for efficacies towards a better future. Furthermore, improving the quality and availability of data plays an important role in creating more diverse datasets beneficially; thus enabling a fair outcome while minimizing bias proportionately.
This better supports ethical considerations comprehensively overlooked otherwise.
Lastly, comprehensive interdisciplinary approaches should be put into practice providing a wider perspective on potential biases or ethical challenges unaccounted for otherwise when developing AI technologies/data analysis frameworks/technologies which fail reproducibility testing/collaborations between researchers with different areas of expertise (e.g., ethics consultants) helps prevent unknown outcomes from arising due to foreseeable limitations in data collection/storage usage algorithms improving accountability measures regarding generating value from investments today helping pave pathways suitably tomorrow!
Emerging research continues on the development of explainable AI techniques – which require interpretable models that can communicate the decision-making process concisely. Despite the recent progress made in this field towards the interpretability of algorithms; regulatory frameworks are lagging behind adequate technological advancement – hence it is important for industry experts worldwide alongside government regulation bodies at large draft inclusive guidelines promoting fairness, and transparency independently within every country or globally as standards.
Conclusion
- It is of utmost importance to prioritize responsible AI in order to maintain impartiality and openness when reporting on data science.
- Algorithms that exhibit bias have the potential to exacerbate discrimination and entrench existing societal disparities.
- Strategies such as diverse and representative data, bias detection and mitigation, interpretable models, and regular auditing promote fairness in AI systems.
- It is crucial to establish transparency in organizational practices. This goal can be achieved by adopting different measures including opening data for sharing, documenting algorithms, defining ethical guidelines, and encouraging public engagement through initiatives.
- Continued efforts are necessary to overcome several hurdles faced in the field. Such challenges include the requirement for establishing standardized evaluation metrics, fostering interdisciplinary collaboration, and creating explainable AI techniques.
- By addressing these challenges, we can build a future where AI is used responsibly, ethically, and for the benefit of all.
- By clicking here, I state that I have read and understood the terms and conditions mentioned above.