Navigate Sharing Your Research Data With DataSeer
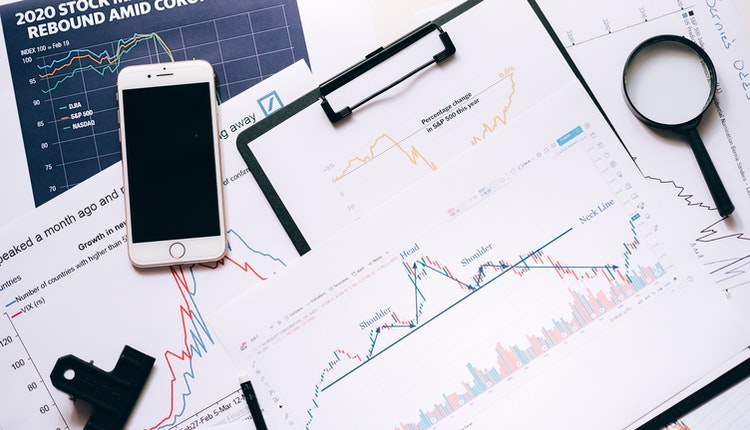
This guest post is drafted by an expert team from DataSeer. It is an intuitive interface that assists in streamlining the process of sharing and preservation of research data.
Science advances on the incremental discoveries of thousands of people working through difficult problems. This advancement is, unfortunately, hampered by a lack of accessible data underlying these research discoveries. Freely accessible data would have the benefit of speeding the rate of discoveries. Furthermore, it would allow for a more thorough understanding of progress than is possible by one group working alone.
In addition, open research data are crucial for public building trust in research – an important consideration in these days of scientific skepticism and misinformation. One of the pillars of the Open Science movement is making data sharing of research articles much more widespread. In fact, many journals and funders have already implemented mandatory data sharing policies. One of the largest challenges with Open Research Data is linking these data sharing requirements to the actions needed by authors to share data for their particular articles.
The Revolutionary DataSeer Approach
DataSeer is an AI app that guides users through the what, how, when, and where of sharing research data. Identifying the data sets in one’s own article seems trivial at first glance! However, it can quickly become complicated when questions of whether sharing raw data is enough, or if the downstream processed data are required instead, or both? This difficulty is greatly magnified if the person assessing data sharing compliance is a journal editor rather than the author. This happens as journal editors lack the authors’ first-hand understanding of what went into the article.
Using Natural Language Processing and textual cues, DataSeer collects and separates sentences that describe data collection. Look below for an example. Furthermore, it infers the type of data being collected, and then identifies the data sets that need to be shared. DataSeer shows authors which data sharing repositories are most appropriate given their circumstances. These may include the type of data, the type of organism they’re working with, and any repository preferences expressed by their journal, institution, or funding agency.
Once authors have been guided through sharing their data on public repositories, DataSeer creates an open certificate. The authors can use this certificate to demonstrate gold-standard compliance with open data policies to other stakeholders, such as their institution or funder. This certification process also generates a permanent, public link between that manuscript and the associated datasets. This step greatly improves the discoverability of both and allowing others to easily track dataset generation and reuse.
How Does DataSeer Achieve All This?
DataSeer has three main parts – the algorithm, the user interface, and the ‘Research Data Wiki’. The DataSeer team has trained the algorithm on over 50,000 sentences from open access articles from journals like PLOS ONE and Scientific Reports. This rigorous approach enables it to handle research articles from a wide range of subject areas. The user interface allows researchers or journals to upload text from articles, and provides a report of the datasets in the article and how to share them. The Wiki hosts best-practice advice for sharing many different types of data. The goal is that widespread use of DataSeer will eventually lead to a global resource on best-practice for data sharing across all areas of research.
Who Does DataSeer Help?
In this age of misinformation and instant sharing, it is key to establish trust in sources and publications. DataSeer provides the pathway for efficiently increasing the proportion of articles that are accompanied by open data. In addition, it aids in increasing the quality and completeness of those open datasets.
Authors and Researchers
DataSeer’s innovation is to use the efficiency of machine learning and natural language processing to automate a really difficult step in enforcing data sharing policies. This includes working out what the authors of a particular article need to do, and helping them do it. At some journals this step is performed by PhD level data curation experts. However, as each article can take them between 30 minutes and an hour to process, this approach is only practical for accepted manuscripts at well-resourced publishers. By making this process much cheaper and quicker, DataSeer will enable many more journals to adopt data sharing policies.
Moreover, because DataSeer is inexpensive and highly scalable, it enables journals to require that all submitted articles share their data, so that the datasets can be scrutinized during peer review. This in turn will prompt researchers to be more rigorous with their data management throughout the research cycle. Furthermore, it should ultimately improve the overall reliability of published work.
DataSeer will also ensure that a much higher proportion of articles share their data, and also do a better job of sharing all of their datasets. Many more datasets will be available for testing new hypotheses, conducting powerful meta-analysis, or just verifying the authors’ results. This is the crux of DataSeer’s innovation: by fixing an apparently minor stumbling block in the peer review process, a revolution in open science is ushered in.
Journals, Publishers, Institutions, & Funding Agencies
Most stakeholders recognize the urgent need to improve the amount and quality of shared research data. Despite their efforts, compliance with data sharing policies (no matter how strongly worded) is frustratingly low. Additionally, the efforts to educate researchers about data sharing and open science are piecemeal and poorly attended. Human curators can ensure that authors of research articles share all of their data. However, since each article requires 15-45 minutes of effort this approach is only available to well-resourced journals. The high-touch human approach is clearly very hard to scale across the c. 2.5 million research articles published each year.
DataSeer’s uses AI and Natural Language Processing to massively scale the demonstrably successful human curation approach. We define the data sharing actions the authors need to take right now for this particular article, lead them through the process, and report on their efforts to the relevant stakeholder. We are collaborating with DataCite to better guide researchers towards the most suitable data repositories. In addition, it will also help them in actively filtering DataSeer’s recommendations according to the journal, author institution, and funding agency associated with a particular article.
The Future of Open Data is Approaching
DataSeer will highlight exactly what needs to be done with authors’ data. By doing so, it will drive a system change in how journals promote Open Research Data. This shall greatly increase both the proportion of articles with open data and the completeness of the datasets shared alongside each article!