How to Share and Reuse Research Data Using FAIR
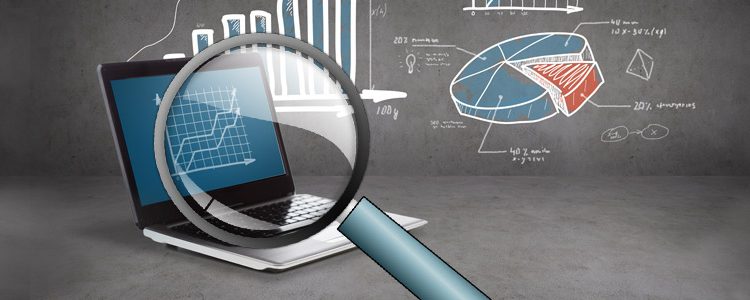
There has been an explosion in the amount of research data generated by the scientists. More and more scientists are tackling complex questions and often the answers can be found using large data sets. This usually requires reusing and sharing research data, making research data management important among researchers. There are several groups working to improve data sharing practices. FORCE11 (the Future of Research Communications and e-Scholarship) is one of them.
FORCE11 was created in 2011. It is a community of scholars, librarians, archivists, publishers, and research funders, focused on improving the way knowledge is created and shared.
Good research data management requires that data are properly collected, annotated, and curated. These steps make it easier for others to find and reuse data. Scientists may choose to analyze the original data or combine it with new or their own data. However, this practice is not that easy. Often, you may need only full data set but also the code or workflow that was originally used with it. This code or workflow may not be publicly available. It is also possible that the research metadata was not properly submitted, making it difficult to interpret the data. There may also be permission or licensing barriers associated with the aces to data.
The FAIR Principle
In an attempt to reduce the difficulty in data sharing, detailed guidelines have been published. In this paper, the FAIR principles have been outlined, supporting data sharing. These guidelines support that data should be FAIR – Findable, Accessible, Interoperable, and Reusable.
Research data needs to be discoverable Few data repositories are already doing a good job of this, for instance, Genbank and the Space Physics Data Facility.
In addition, open access data repositories such as FigShare, Dryad, Zenodo, and DANS host different data types and formats. Such repositories do not typically try to harmonize or integrate the archived data. This means that data is not centralized, making it harder for researchers to find what they need.For data to be accessible, it should be easily downloadable in a format that does not require any obscure software to open it. It should be easy for a researcher not only to download the data but also to integrate it with their own data.
Interoperability means that the data exist in a format that can be widely used. The data must use shared technologies and standards. The alternative would mean creating custom parsers for each data types, in all computer languages, and for all the associated analytical tools. This would be tedious.
Finally, the data must be reusable. Licensing information should be available. This will help scientists (and machines) to determine how the data can be used. The data should be richly annotated. There should be detailed information about the source of the data as well.
FAIR Compliance
Improving the reusability of data will require some effort. The publication includes a minimum requirements checklist, make it easier for to comply with the guidelines. This list can guide researchers as they prepare to share their data. The checklist has requirements for both data and metadata.
The guidelines include requirements such as:
- Data should have a unique and persistent identifier
- Data should be described by rich metadata
- Data and metadata should be indexed or registered in a searchable resource
- Data and metadata should be retrievable by their unique identifier via a standard communications protocol
- This protocol should be open, free, and universally implementable
- The protocol should make authentication and authorization possible as needed
- Data and metadata should use a formal, accessible, and broadly applicable language to represent knowledge
- Data and metadata should use vocabularies that comply with FAIR principles
- Data and metadata should be richly described with many accurate and relevant attributes
- Data and metadata should be released with a clear and accessible data usage license
- Data and metadata should meet domain-relevant community standards.
The Data Citation Implementation Group of FORCE11 has published recommendations for implementing these principles. The FAIR principles make data sharing easier. As more scientists adhere to these principles, research data will be easier to find, access, and reuse. It will also be easier to mine existing data for new insights.