R-Factor to Reduce Instances of Scientific Fraud?
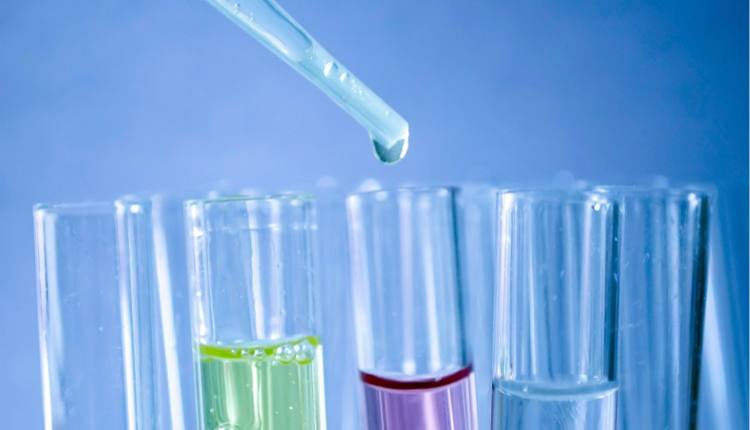
A significant chunk of research published in the last few years has faced reproducibility issues. According to a recent report, only 6 out of 53 landmark cancer studies were found to be reproducible. This makes us question the value of journal articles. How can we trust a scientific finding that simply cannot be confirmed? There needs to be something apart from journal ranking to indicate the quality of published research. This is where the R-factor comes into picture. It offers a way to assess journal articles based on their reproducibility.
The researchers who came up with the concept of R-factor hope that it will discourage scientists from publishing fraudulent data. They also hope that it will increase the actual publication of negative results. The R-factor can be calculated for a paper, a researcher, or an institution and can change over time. It is a dynamic way of confirming the reliability of scientists and their published papers.
The R-factor is easy to calculate and ranges from zero to one. It is simply a ratio of the number of papers that support the original claim to the number of papers that tested it. Thus, a low R-factor research article published in a prestigious journal could be quite embarrassing to the concerned researchers. Because negative results are needed to determine the true R-factor of a paper, more scientists might publish such results.
Replication studies have traditionally been the best way to test reproducibility. However, this is an expensive and time-consuming process. There is also very little reward offered to scientists for replication studies and there is no prestige associated with it. Replication studies also lack novelty and do not help scientists advance in their careers.
The R-factor can be calculated at a low cost and it introduces a level of accountability. It shifts focus from merely publishing journal articles to making sure the paper can stand the test of time. In the current system, it is impossible to keep up with all the journal articles being published. Unless a scientist is accused of fraud, there are no penalties for publishing flawed data. The R-factor would place every article under greater scrutiny.
R-Factor Limitations
Details pertaining to the R-factor are available on biorXiv. The R-factor is based on published reports and does have some problems. We know that publication bias affects research literature as the publication of positive results is more favored over the publication of negative results. The R-factor does not distinguish between a good paper and a bad paper. If a paper supports a previous claim by p-hacking, it would still be used to calculate the R-factor. This would immediately skew the results.
When calculated, the R-factor treats all papers as equal. However, this assumption is not always valid. Larger studies generally are more trustworthy than smaller ones. It could also be difficult to decide whether a study confirms a claim or not. The data in a journal article may strongly support previous work, be inconclusive, or be everything in between.
The R-factor’s simplicity may also contribute to its downfall. In the case of more complex phenomena, it could be difficult to decide what it takes to confirm a finding. Let’s say we wanted to calculate the R-factor for a journal article that claims that antidepressant consumption leads to suicide. We find a paper claiming that antidepressants increase suicide attempts but not actual deaths. You could say that the paper refutes the original claim if attempted suicides don’t count. However, it would be foolish to ignore the association between antidepressants and suicide attempts.
The R-factor itself doesn’t take the number of papers testing the original claim into account. This means that a paper can have a high R-factor because very few papers have tested its claim and most of them confirm the original finding. Therefore, it is important to add a subscript while reporting the R-factor. This subscript will indicate how many papers have tested the paper’s claim.
Will It Be Useful?
The R-factor has the advantage of being easy to understand. However, calculating it can be difficult, because you first need to read all the papers that tested the original claim. The inventors of the R-factor have manually calculated this journal metric for 12,000 papers. They are hoping to transition to a machine-based learning system to automate the process. The R-factor has obvious shortcomings as it assumes all papers to be equal. It is subject to publication bias. However, it could encourage authors to publish more robust data, by acting as a psychological deterrent.
There is no doubt that we need to improve reproducibility in academic research. The R-factor could provide an easy way to decide the value of journal articles. A journal’s rank is not the measure of a paper. Moreover, the publication of good research work does not always happen only in prestigious journals. The R-factor is a new quality metric that uses other journal articles to calculate the reproducibility of a claim. Is it robust enough to be truly useful to the scientific community? Only time will tell.
Do you think this new metric will address the reproducibility crisis? Will it act as a psychological deterrent? Please share your thoughts by adding your comments below.